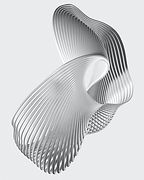
Navigating the AI Revolution in Health Science: Balancing Innovation with
Ethical Governance
Author: Stephen Dela Ahator, 2024
Vision for the Future.
The future of AI in healthcare is auspicious. AI has the potential to revolutionize personalized and precise treatments, discover new therapies, and combat antibiotic resistance leading to the improvement of human lives. However, this future must be built on a foundation of thoughtful governance. As AI becomes more deeply embedded in health research, we must continue to refine and adapt our governance models to keep pace with technological advancements. This includes ensuring that AI systems are developed inclusively, addressing the needs of diverse populations, and minimizing the risk of bias or inequality in healthcare outcomes. By embracing a collaborative and forward-thinking approach, we can ensure that AI in health research not only pushes the boundaries of what is possible but does so in a way that is safe, ethical, and equitable for all.
Abstract
Artificial Intelligence (AI) is revolutionizing health science research by enabling machines to mimic human cognitive processes, analyze large datasets, and uncover complex biological patterns that enhance evidence-based decision-making. This advancement has transformed research methodologies and accelerated innovation across various scientific disciplines, where AI excels in tasks like medical image analysis, biological data pattern recognition, biomarker identification and clinical decision-making. However, as AI becomes more integrated into health science, the need for robust governance frameworks is critical to managing associated risks. Without proper governance, AI could perpetuate societal biases, compromise participant privacy, and undermine trust in health research. Effective AI governance must ensure transparency, fairness, and accountability, particularly in addressing biases that could lead to biased research outputs. Current regulatory frameworks, such as GDPR provide a foundation for data protection but are not fully equipped to handle the complexities of AI advancement. Harmonizing AI regulations globally and developing adaptive frameworks are essential to keeping pace with rapid technological advancements. The future of AI in health research is promising. However, realizing this potential responsibly requires a commitment to thoughtful governance. By encouraging ongoing dialogue among stakeholders and balancing innovation with safety, we can ensure that AI in health science is developed and deployed in a way that is ethical, inclusive, and equitable for all.
The Role of AI in Health Science Research
AI's Transformative Potential. AI has emerged as a transformative research paradigm, enabling machines to mimic human thought processes like reasoning, prediction, and decision-making. It allows for the development of intelligent algorithms and programs that can efficiently perform tasks typically requiring skilled human expertise. (Collins et al., 2021; Rawas, 2024; Xu et al., 2021). This has fundamentally changed research methodologies, enabling the analysis of large datasets and the discovery of hidden patterns in biological systems. In health science research, AI is revolutionizing the field by processing vast amounts of data quickly and accurately, revealing complex patterns and insights that would be challenging and time-consuming for humans to detect(Alowais et al., 2023). Additionally, AI has accelerated research across various scientific disciplines, integrating them seamlessly into health science, driving innovation, and advancing applied research platforms. AI, particularly deep learning, has demonstrated exceptional performance in analyzing medical images, often surpassing human accuracy (Alowais et al., 2023; Xu et al., 2021). It automates critical aspects of cancer diagnosis, such as analyzing pathology slides and detecting tumour-infiltrating lymphocytes. AI-driven tools like the molecular Prognostic Score predict patient outcomes more accurately than traditional methods, leading to more effective treatment decisions(Alowais et al., 2023; Peng et al., 2021).
The Need for Governance
As AI becomes more integrated into health science research, it brings significant transformative potential but also considerable risks without proper management. AI governance is crucial for guiding the responsible development, deployment, and use of AI, particularly in health research, where the stakes are high. AI systems are often trained on large datasets that may contain biases reflecting existing societal inequalities, leading to decisions that can profoundly impact patient outcomes(McKay et al., 2022; Roski et al., 2021).
Without proper governance, the risks associated with AI such as misuse, bias, and ethical breaches can escalate, potentially amplifying these biases, which could result in unfair treatment of certain groups based on race, gender, or socioeconomic status(Organization, 2024a). Moreover, AI systems handle large and sensitive data, including personal health information. Without stringent governance, the risk of privacy violations, data breaches, and unethical data use increases, potentially eroding public trust in AI technologies and research outputs(Ferrara, 2023; Mensah, 2023).
Additionally, as AI systems advance, they can operate as "black boxes," making decisions that are not easily understood by humans (Hassija et al., 2024). Implementation of critical governance frameworks will ensure that these technologies are developed ethically, transparently, and in alignment with societal values. Moreover, the frameworks should address the challenges posed by AI's ability to process vast amounts of data and make autonomous decisions, which can lead to unintended consequences if not properly managed. The autonomous nature of AI raises questions about accountability and the potential loss of human oversight in critical decision-making processes. Without governance, AI might prioritize efficiency or profitability over patient safety and ethical considerations, leading to negative outcomes for individuals and society (Ferrara, 2023; Mensah, 2023; Safdar et al., 2020; Taeihagh, 2021). Proper AI governance is essential for mitigating risks, ensuring fairness and equity, and maintaining public trust in these technologies, particularly as AI is increasingly used to analyze complex data, diagnose conditions, and make inferences on research data (Mensah, 2023)

Ethical Considerations
AI systems often learn from historical data that reflects existing societal biases. When these biases are embedded in the training data, AI models risk not only replicating but also amplifying these disparities(Ferrara, 2023; Mensah, 2023; Organization, 2024b).
​
Similarly, a convolutional neural network developed to detect left ventricular ejection fraction from ECG data, initially trained on a predominantly non-Hispanic white population, underscores the importance of rigorously testing AI models across diverse groups (Khunte et al., 2023; Noseworthy et al., 2020). Without such testing, AI models may perform poorly for underrepresented demographics, leading to misdiagnoses or suboptimal treatment recommendations. Thus, to ensure fairness and equity, AI governance must ensure all AI models undergo rigorous bias testing, including analyzing training data for fairness, applying bias mitigation techniques during development, and continuously monitoring systems post-deployment. Models should be trained on data representative of all population groups, requiring intentional inclusion of underrepresented demographics to prevent bias (Alvarez et al., 2024). Transparency in AI decision-making is also crucial, ensuring algorithms are understandable to stakeholders and that accountability for decisions is maintained.
AI systems in health research involving clinical studies must operate transparently, especially in decision-making, to maintain patient autonomy. Patients need to understand how AI influences their diagnosis and treatment. The complexity of AI can complicate informed consent, as patients may not fully grasp how algorithms work, leading to potential misunderstandings or mistrust. For informed consent to be meaningful, patients must be aware of how AI is applied, what data it uses, and how biases might impact their care.
Using clinical data in AI systems often raises significant privacy and data security concerns. Integrating AI into clinical research involves handling vast amounts of personal health information, which, if not securely managed, can lead to data breaches (Frank & Olaoye, 2024; Murdoch, 2021; Redrup Hill et al., 2023). Enforcing strict data security protocols to prevent misuse and unauthorized access is vital. Additionally, obtaining explicit patient consent for data use in AI systems is essential to maintaining privacy and upholding ethical standards in health research(Frank & Olaoye, 2024)(Khalid et al., 2023)
Balancing Innovation with Safety
​
AI governance frameworks that encourage innovation while ensuring safety has tremendous potential to revolutionize diagnosis, treatment, and research outcome. However, a significant challenge lies in designing regulatory frameworks that do not stifle this innovation. Governance frameworks should be constructed to support AI developers and researchers in exploring new AI applications. This includes providing flexibility within regulations to allow for the rapid evolution of AI technologies, ensuring that innovation is not unduly hampered by overly rigid or outdated rules.
The management of risks associated with AI in the health sciences is critical to balancing innovation with safety. AI systems, particularly in clinical research, come with inherent risks such as algorithmic errors, and complex liability issues. A structured governance model can help mitigate these risks by providing clear guidelines on the development, testing, and deployment of AI technologies. This includes establishing liability frameworks that ensure that all stakeholders, including developers, healthcare providers, and manufacturers, are accountable for the safe use of AI systems.
AI Governance Models and Best Practices
​
The pragmatic approach in open science advocates for collaboration between diverse stakeholder groups, such as citizens, academics, practitioners, and policymakers. This collaboration is essential for addressing complex ethical challenges in AI governance, ensuring that a wide range of perspectives are considered in decision-making processes (Saheb & Saheb, 2024). Effective AI governance involves the participation of multiple stakeholders to shape policies that are inclusive and reflective of diverse societal values. Involving a diverse array of stakeholders in AI governance helps to ensure that the systems developed are fair, equitable, and sensitive to the needs of different demographic groups. For example, engaging underrepresented communities in the development process can help identify and mitigate potential biases in AI algorithms. Multi-stakeholder involvement is also crucial for fostering trust in AI technologies.
​
Transparency in AI development is crucial for building trust and ensuring accountability. To ensure that AI systems are transparent and accountable, it is essential to incorporate explainability into the design of AI models (Balasubramaniam et al., 2023). This means that AI systems should be able to provide clear, understandable explanations for their decisions, which can be scrutinized by users, regulators, and the public. Accountability in AI governance can be strengthened by establishing clear roles and responsibilities for all stakeholders involved in the development and deployment of AI systems. This includes legal frameworks that define liability and mechanisms for redress in cases where AI systems cause harm.
​
Open science practices, such as open data sharing and collaborative research, are critical for ensuring that AI development is transparent and inclusive (Ng et al., 2024). Open science in the context of AI governance involves making research data and findings accessible to a broad audience, facilitating collaboration and ensuring that AI systems are developed ethically and responsibly. By sharing data openly, researchers and developers can work together to improve AI models, making them more accurate and less biased. However, open data sharing must be managed carefully to protect sensitive information
The Need for Governance
As AI becomes more integrated into health science research, it brings significant transformative potential but also considerable risks without proper management. AI governance is crucial for guiding the responsible development, deployment, and use of AI, particularly in health research, where the stakes are high. AI systems are often trained on large datasets that may contain biases reflecting existing societal inequalities, leading to decisions that can profoundly impact patient outcomes(McKay et al., 2022; Roski et al., 2021).
Without proper governance, the risks associated with AI such as misuse, bias, and ethical breaches can escalate, potentially amplifying these biases, which could result in unfair treatment of certain groups based on race, gender, or socioeconomic status(Organization, 2024a). Moreover, AI systems handle large and sensitive data, including personal health information. Without stringent governance, the risk of privacy violations, data breaches, and unethical data use increases, potentially eroding public trust in AI technologies and research outputs(Ferrara, 2023; Mensah, 2023).
Additionally, as AI systems advance, they can operate as "black boxes," making decisions that are not easily understood by humans (Hassija et al., 2024). Implementation of critical governance frameworks will ensure that these technologies are developed ethically, transparently, and in alignment with societal values. Moreover, the frameworks should address the challenges posed by AI's ability to process vast amounts of data and make autonomous decisions, which can lead to unintended consequences if not properly managed. The autonomous nature of AI raises questions about accountability and the potential loss of human oversight in critical decision-making processes. Without governance, AI might prioritize efficiency or profitability over patient safety and ethical considerations, leading to negative outcomes for individuals and society (Ferrara, 2023; Mensah, 2023; Safdar et al., 2020; Taeihagh, 2021). Proper AI governance is essential for mitigating risks, ensuring fairness and equity, and maintaining public trust in these technologies, particularly as AI is increasingly used to analyze complex data, diagnose conditions, and make inferences on research data (Mensah, 2023)
Regulatory Challenges
In Europe, the General Data Protection Regulation (GDPR) is a key legal framework focused on data protection and privacy, requiring explicit consent for data processing, stringent protection measures, and ensuring data portability. However, GDPR is not specifically tailored to AI's unique challenges, such as the need for transparency in algorithmic decision-making and handling large, complex research datasets (Regulation, 2016). Its primary limitation is that it wasn't designed with AI in mind, which may leave gaps in addressing AI-specific issues like algorithmic transparency, bias management, and ethical guidelines for AI in healthcare.
​
Considering the regulatory landscape across borders is fragmented, with varying standards and practices. International collaboration is essential to harmonize AI regulations, ensuring consistent, ethical, and effective development and use. This harmonization requires a common framework addressing key issues like data protection, AI transparency, algorithmic fairness, and accountability. Also, the unprecedented pace of AI technology advancement often outstrips the ability of regulatory frameworks to keep up. This rapid innovation can lead to regulatory gaps, where existing laws are insufficient to address new technologies and their potential risks (Walter, 2024). To address the challenge, regulatory frameworks must be flexible and adaptive. This means moving away from rigid, prescriptive regulations and towards frameworks that can evolve alongside technological advancements. Adaptive regulations would also involve close collaboration between regulators (Aquino et al., 2024), AI developers, healthcare providers, funding bodies for human health researchers and other stakeholders. By working together, these groups can identify emerging risks, share best practices, and develop guidelines that reflect the current state of AI technology.
Future Directions
As AI technology evolves, emerging challenges will require governance frameworks to adapt. Using AI to tailor health science research based on genetic, behavioral, and environmental data presents privacy, informed consent, and bias concerns that could lead to unequal treatment(Alvarez et al., 2024; Frank & Olaoye, 2024). Governance models must evolve to ensure research advancements using AI in personalized medicine, disease biomarker discovery or predicting disease outbreaks is both effective and ethical. governance models must continuously assess and update regulatory frameworks to remain relevant amid rapid technological advancements. AI itself could shape and enforce governance, providing real-time oversight and adaptive regulation. This includes designing AI to monitor compliance, detect biases, ensure transparency, and identify deviations from ethical guidelines. By overseeing AI with AI, governance frameworks can become more responsive to emerging issues.
​
The path forward.
A key aspect of moving forward is fostering an ongoing dialogue among researchers, clinicians, policymakers, ethicists, and stakeholders involved in AI development and application(Alvarez et al., 2024; Aquino et al., 2024). These discussions are crucial for understanding the diverse perspectives and needs that must be addressed in AI governance. Collaboration across these groups will lead to the creation of governance frameworks that are effective in mitigating risks and flexible enough to adapt to the fast-paced advancements in AI technology(Board, 2024). By working together, stakeholders can ensure that AI not only accelerates the progress of health science research but does so in a way that aligns with ethical principles and societal values.
References
Alowais, S. A., Alghamdi, S. S., Alsuhebany, N., Alqahtani, T., Alshaya, A. I., Almohareb, S. N., Aldairem, A., Alrashed, M., Bin Saleh, K., & Badreldin, H. A. (2023). Revolutionizing healthcare: the role of artificial intelligence in clinical practice. BMC Medical Education, 23(1), 689.
​
Alvarez, J. M., Colmenarejo, A. B., Elobaid, A., Fabbrizzi, S., Fahimi, M., Ferrara, A., Ghodsi, S., Mougan, C., Papageorgiou, I., & Reyero, P. (2024). Policy advice and best practices on bias and fairness in AI. Ethics and Information Technology, 26(2), 31.
​
Aquino, Y. S. J., Rogers, W. A., Jacobson, S. L. S., Richards, B., Houssami, N., Woode, M. E., Frazer, H., & Carter, S. M.(2024). Defining change: Exploring expert views about the regulatory challenges in adaptive artificial intelligence for healthcare. Health Policy and Technology, 13(3), 100892. https://doi.org/https://doi.org/10.1016/j.hlpt.2024.100892
​
Balasubramaniam, N., Kauppinen, M., Rannisto, A., Hiekkanen, K., & Kujala, S. (2023). Transparency and explainability of AI systems: From ethical guidelines to requirements. Information and Software Technology, 159, 107197. https://doi.org/https://doi.org/10.1016/j.infsof.2023.107197
​
Board, C. E. (2024). United Nations System White Paper on AI Governance. Policy.
​
Collins, C., Dennehy, D., Conboy, K., & Mikalef, P. (2021). Artificial intelligence in information systems research: A systematic literature review and research agenda. International Journal of Information Management, 60, 102383. https://doi.org/https://doi.org/10.1016/j.ijinfomgt.2021.102383
​
Ferrara, E. (2023). Fairness and bias in artificial intelligence: A brief survey of sources, impacts, and mitigation strategies. Sci, 6(1), 3.
​
Frank, E., & Olaoye, G. (2024). Privacy and data protection in AI-enabled healthcare systems.
​
Hassija, V., Chamola, V., Mahapatra, A., Singal, A., Goel, D., Huang, K., Scardapane, S., Spinelli, I., Mahmud, M., & Hussain, A. (2024). Interpreting Black-Box Models: A Review on Explainable Artificial Intelligence. Cognitive Computation, 16(1), 45–74. https://doi.org/10.1007/s12559-023-10179-8
​
Khalid, N., Qayyum, A., Bilal, M., Al-Fuqaha, A., & Qadir, J. (2023). Privacy-preserving artificial intelligence in healthcare: Techniques and applications. Computers in Biology and Medicine, 158, 106848.
https://doi.org/https://doi.org/10.1016/j.compbiomed.2023.106848
​
Khunte, A., Sangha, V., Oikonomou, E. K., Dhingra, L. S., Aminorroaya, A., Mortazavi, B. J., Coppi, A., Brandt, C. A., Krumholz, H. M., & Khera, R. (2023). Detection of left ventricular systolic dysfunction from single-lead electrocardiography adapted for portable and wearable devices. Npj Digital Medicine, 6(1), 124. https://doi.org/10.1038/s41746-023-00869-w
​
McKay, F., Williams, B. J., Prestwich, G., Treanor, D., & Hallowell, N. (2022). Public governance of medical artificial intelligence research in the UK: an integrated multi-scale model. Research Involvement and Engagement, 8(1), 21.Mensah, G. B. (2023). Artificial intelligence and ethics: a comprehensive review of bias mitigation, transparency, and accountability in AI Systems. Preprint, November, 10.
​
Murdoch, B. (2021). Privacy and artificial intelligence: challenges for protecting health information in a new era. BMC Medical Ethics, 22(1), 122. https://doi.org/10.1186/s12910-021-00687-3
Ng, J. Y., Wieland, L. S., Lee, M. S., Liu, J., Witt, C. M., Moher, D., & Cramer, H. (2024). Open science practices in traditional, complementary, and integrative medicine research: A path to enhanced transparency and collaboration. Integrative Medicine Research, 13(2), 101047. https://doi.org/https://doi.org/10.1016/j.imr.2024.101047
​
Noseworthy, P. A., Attia, Z. I., Brewer, L. C., Hayes, S. N., Yao, X., Kapa, S., Friedman, P. A., & Lopez-Jimenez, F. (2020). Assessing and mitigating bias in medical artificial intelligence: the effects of race and ethnicity on a deep learning model for ECG analysis. Circulation: Arrhythmia and Electrophysiology, 13(3), e007988.
​
Organization, W. H. (2024a). Ethics and governance of artificial intelligence for health: large multi-modal models. WHO guidance. World Health Organization.
​
Organization, W. H. (2024b). Ethics and governance of artificial intelligence for health: large multi-modal models. WHO guidance. World Health Organization.
​
Peng, Q., Shen, Y., Fu, K., Dai, Z., Jin, L., Yang, D., & Zhu, J. (2021). Artificial intelligence prediction model for overall survival of clear cell renal cell carcinoma based on a 21-gene molecular prognostic score system. Aging (Albany NY), 13(5), 7361.
​
Rawas, S. (2024). AI: the future of humanity. Discover Artificial Intelligence, 4(1), 25. https://doi.org/10.1007/s44163-024-00118-3
​
Redrup Hill, E., Mitchell, C., Brigden, T., & Hall, A. (2023). Ethical and legal considerations influencing human involvement in the implementation of artificial intelligence in a clinical pathway: A multi-stakeholder perspective. Frontiers in Digital Health, 5, 1139210.
​
Regulation, P. (2016). Regulation (EU) 2016/679 of the European Parliament and of the Council. Regulation (Eu), 679, 2016.
Roski, J., Maier, E. J., Vigilante, K., Kane, E. A., & Matheny, M. E. (2021). Enhancing trust in AI through industry self- governance. Journal of the American Medical Informatics Association, 28(7), 1582–1590. https://doi.org/10.1093/jamia/ocab065
​
Safdar, N. M., Banja, J. D., & Meltzer, C. C. (2020). Ethical considerations in artificial intelligence. European Journal of Radiology, 122, 108768.
​
Saheb, T., & Saheb, T. (2024). Mapping Ethical Artificial Intelligence Policy Landscape: A Mixed Method Analysis. Science and Engineering Ethics, 30(2), 9.
​
Taeihagh, A. (2021). Governance of artificial intelligence. Policy and Society, 40(2), 137–157. https://doi.org/10.1080/14494035.2021.1928377
​
Walter, Y. (2024). Managing the race to the moon: Global policy and governance in Artificial Intelligence regulation—A contemporary overview and an analysis of socioeconomic consequences. Discover Artificial Intelligence, 4(1), 14. https://doi.org/10.1007/s44163-024-00109-4
​
Xu, Y., Liu, X., Cao, X., Huang, C., Liu, E., Qian, S., Liu, X., Wu, Y., Dong, F., & Qiu, C.-W. (2021). Artificial intelligence: A powerful paradigm for scientific research. The Innovation, 2(4).
​
Xu, Y., Liu, X., Cao, X., Huang, C., Liu, E., Qian, S., Liu, X., Wu, Y., Dong, F., Qiu, C.-W., Qiu, J., Hua, K., Su, W., Wu, J., Xu, H., Han, Y., Fu, C., Yin, Z., Liu, M., … Zhang, J. (2021). Artificial intelligence: A powerful paradigm for scientific research. The Innovation, 2(4), 100179. https://doi.org/https://doi.org/10.1016/j.xinn.2021.100179